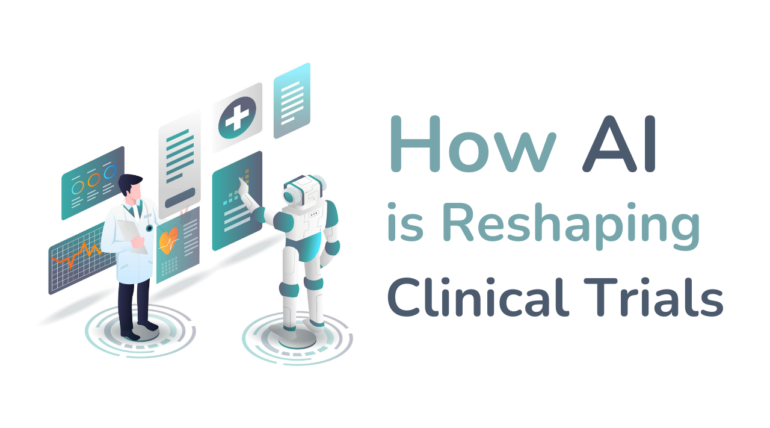
Research and invention play an increasingly prominent role in the growth of any industry. Nonetheless, in medical care, these trials and researches are carried out to proceed with the new treatments, drugs, and solutions for the ever-evolving and foregoing diseases.
New drug testing and analysis is a time-consuming and expensive process. Besides stricter regulatory standards and greater emphasis on the safety of patients and trial oversight, the clinical development marketplace is becoming more competitive.
But Artificial Intelligence-powered technology can alter every phase of the clinical trial process from patient recruitment to adherence monitoring and data collection. It is driving trial efficiencies by improving end-to-end clinical trial processes and assisting pharma companies to bring brand new drugs and therapies to the market quicker. Let us have a look at some applications of AI-enabled technology in clinical trials:
Clinical trial design:
Pharma companies are adopting a range of methods to access large amounts of scientific and research data, including a variety of sources, but have often lacked the ability and technologies to efficiently utilize this data. The scientific and research data, which includes past and current clinical trials, post-market surveillance, case studies of special medical situations, and patient support programs, etc. have refueled the trial designs. AI-powered technologies have a unique potential to gather, analyze, and organize this data and draw significant patterns of data to greatly help with the design.
Additionally, such algorithms can find the best-suited scenarios and timings for the trials, which can also help to get over the drawbacks of past trials.
Patient recruitment, and enrichment:
With roughly 30% of patients dropping away from clinical studies and nearly half of all investigative websites under-enrolling, patient retention and recruitment are among a few challenges that the clinical industry is facing today. AI can help pharma deal with both these challenges, thereby enhancing patient selection and clinical trial effectiveness. This can be done through mining, interpretation and analysis of multiple data sources, which is further associated with omics data, medical imaging, and electronic health records (EHRs). This data is used and assessed by AI to determine a person suitable for clinical trials, which further helps in reducing population heterogeneity, which is recommended to lower variability in advance, thereby increasing the study power.
Also, AI systems are capable of performing (i) prognostic enrichment to identify patients with considerable clinical endpoints, and (ii) predictive enrichment to identify the patients most likely to respond to the treatment, thereby ensuring that, as per the criteria, only the most suitable patients take part in the trials.
Investigator and site selection:
AI programs can suggest appropriate sites and locations for the testing based on the geographical and climatic conditions of the area. It is one of the most important aspects of the trial, which includes proper availability of resources and an adequate number of professionals with in-depth knowledge of the disease to attend the patients and emergency facilities. Additionally, advanced analytics techniques offer the best solutions for finding an appropriate site for performing clinical tests by integrating themselves with population count and map data.
Patient monitoring, medication adherence, and retention:
AI algorithms use the data to monitor the post-trial and pre-trial conditions of patients. Alongside the effective monitoring, real-time safety monitoring is performed with the proper assessment of the risk. This can be done by using sensor-based wearable products during the trials. Furthermore, an AI-based system connected to the devices automatically records any change in body temperature or pulse if detected all throughout the trial and also the change in a few hours after giving the drug. In the case of further research and analysis with fewer possibilities of discrepancy, this data can be transferred across the system in almost no time using AI.
AI makes clinical trials intelligent:
Furthermore, AI can offer pharmaceutical researchers extra predictive information that can help to determine whether taking a drug will have a positive or negative effect, whether patients will back out from studies, and whether trials will likely be successful or not. Making use of AI techniques and prognostic algorithms, trial risks are reduced well before they become a major problem, thereby maintaining trials on track as well as on budget.
Fostering more trials:
A large amount of data is created during the trials of the medicines, which is shared with other companies whenever they execute the same procedures. In these circumstances, Artificial Intelligence allows sharing the data with other companies to assist them in getting insights about the different criteria that might be followed in their research and create collective data for a similar clinical testing procedure that can prove to be more comprehensive and better.
Conclusion:
Clinical Trials are more of a research-based technique that generates a large amount of data. Mostly, this is a very time-consuming process with no alternative. Significantly, less than 10% of clinical trials end on time and the costs to develop new drugs are sky-rocketing. However, artificial intelligence (AI) can assist in reversing these tendencies, allowing sponsors to improve clinical trials and speed up new product development. Additionally, AI has the ability to disrupt each and every stage of the clinical trial process, from maximizing patient enrollment and retention to enhancing data collection and risk tracking, thereby helping improve its efficiency by providing the right solutions and improvisations by assessing the data.
Researchers incorporating these technologies can significantly reduce the time it takes to promote life-saving drugs and deliver a big victory for the patients who need them most.